Pharmacovigilance is a crucial part of the healthcare system, working tirelessly behind the scenes to ensure that the medications we rely on are safe and effective. By monitoring, assessing, and understanding the side effects and adverse reactions of pharmaceuticals, pharmacovigilance aims to minimize risks associated with drug use. This system is essential in safeguarding patient health by keeping a vigilant eye on any unexpected issues that might arise from drug consumption.
Challenges in Traditional Pharmacovigilance
Despite its importance, traditional pharmacovigilance comes with its share of challenges. Labor-intensive data collection and analysis processes often slow down the identification of potential safety concerns. Delays in data reporting and limited access to diverse, real-world patient information can further hinder the speed at which safety issues are detected. Additionally, relying heavily on voluntary reporting from healthcare professionals and patients means that many adverse effects may go unnoticed, leaving gaps in drug safety measures.
Importance of Proactive Drug Safety Measures
Ensuring proactive drug safety measures means going beyond merely reacting to issues after they occur. With ever-increasing complexities in drug development and diverse patient populations, the need for early detection and prevention of drug safety problems is more critical than ever. Proactive measures can help anticipate potential risks, allowing healthcare providers to mitigate these dangers before they affect patient health. By being forward-thinking, the healthcare industry can provide safer and more reliable treatment options for everyone.
Integration of Artificial Intelligence in Pharmacovigilance
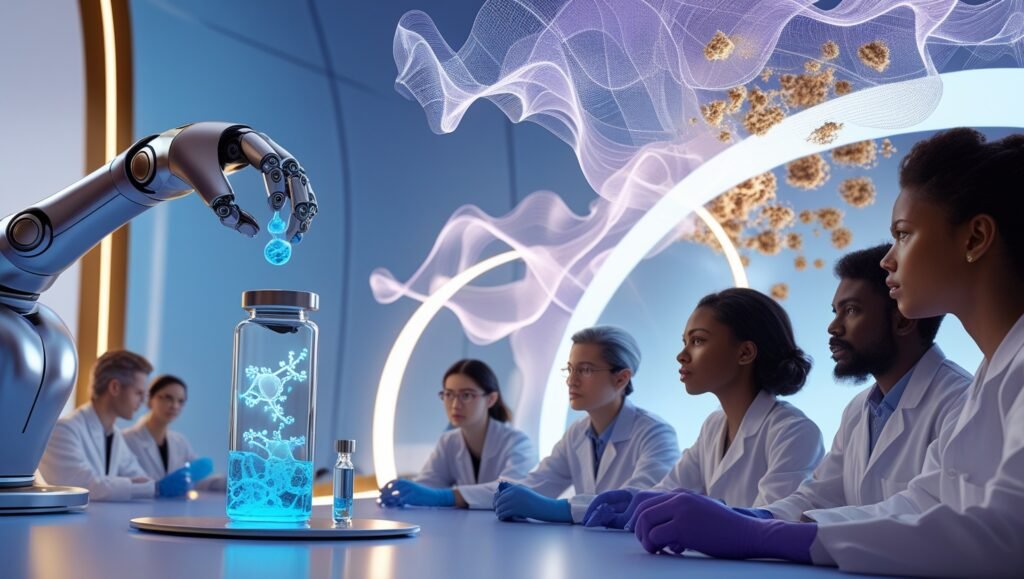
With the evolution of technology, pharmacovigilance has embraced artificial intelligence (AI) to revolutionize its processes. AI’s ability to analyze vast volumes of data quickly and accurately presents new opportunities in drug safety monitoring. Let’s dive into how AI is becoming a key player in enhancing pharmacovigilance.
AI Technologies Used in Pharmacovigilance
Several AI technologies are now at the forefront of pharmacovigilance efforts:
– Machine Learning (ML): This aspect of AI involves training algorithms to recognize patterns within complex datasets. In pharmacovigilance, ML can rapidly identify potential drug safety signals by sifting through vast amounts of data from various sources, including clinical trial results, electronic health records, and patient reports.
– Natural Language Processing (NLP): NLP helps in extracting and interpreting unstructured data from text sources such as social media posts, scientific literature, and medical records. This technology can detect early warning signs from patient feedback and adverse event reports, which might otherwise go unnoticed.
– Robotic Process Automation (RPA): RPA increases efficiency by automating routine tasks such as data entry and initial data processing. With RPA, organizations can ensure that human resources focus on more complex analyses and decision-making.
Benefits of AI in Enhancing Drug Safety
The integration of AI in pharmacovigilance offers numerous benefits that go beyond traditional methods.
1. Faster Detection: AI can monitor real-time data streams, promptly identifying potential drug safety issues that need immediate attention. By shortening the time to detect adverse events, AI helps in initiating quicker interventions to minimize patient risk.
2. Improved Efficiency: AI models streamline processes by handling large datasets and reducing human error. As a result, resources are redirected to areas that necessitate human expertise, such as regulatory communication and detailed case analysis.
3. Cost-effectiveness: While initial investments in AI technology might be significant, the long-term savings from increased process efficiency and better resource allocation make AI cost-effective. Reduced adverse drug events also translate to savings in healthcare costs.
4. Enhanced Data Insights: AI enables deeper analysis of pharmacovigilance data, uncovering hidden correlations and risk factors. These insights can inform drug development, leading to safer pharmaceuticals and personalized medicine approaches.
Case Studies of AI Application in Pharmacovigilance
Numerous real-world examples highlight the efficacy of AI in the field of pharmacovigilance:
– IBM Watson and Pfizer Collaboration: By harnessing Watson’s cognitive computing capabilities, Pfizer streamlined its drug safety monitoring operations. AI helps to process and analyze scientific papers, clinical trial reports, and patient records, identifying critical safety data that could otherwise be missed.
– FDA’s Sentinel Initiative: The U.S. Food and Drug Administration employs AI tools for the Sentinel Initiative to enhance drug safety surveillance. AI helps to analyze electronic health data, contributing to earlier detection of medication safety issues.
– Bayer’s Pharmacovigilance System: Bayer uses AI solutions to read and interpret adverse event reports. Through machine learning models, the company has improved its ability to identify significant safety signals and react more swiftly.
Predictive Analysis with AI
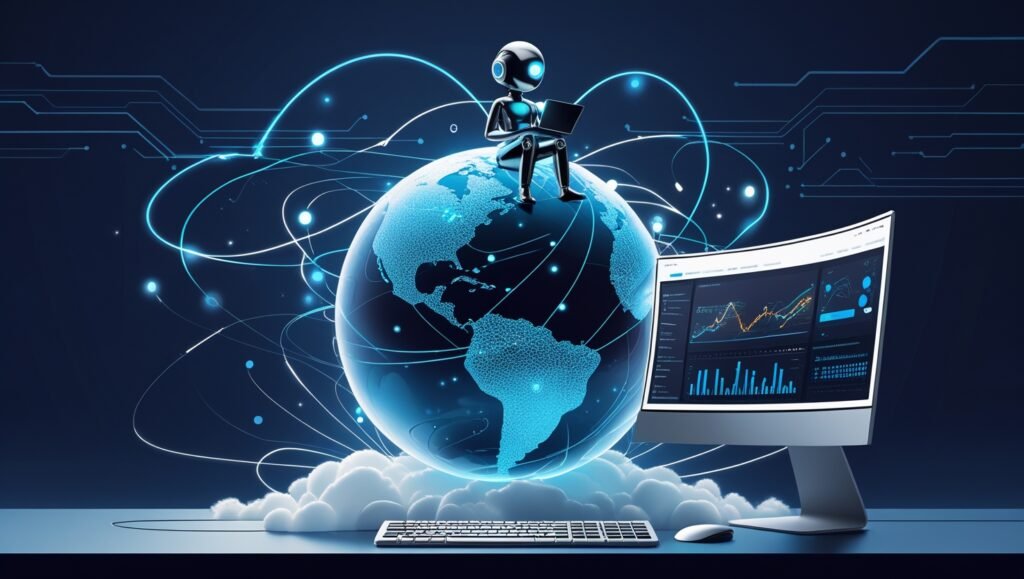
Predictive analysis involves using data, algorithms, and machine learning techniques to identify the likelihood of future outcomes based on historical data. In the domain of pharmacovigilance, AI enables predictive analysis to forecast and prevent adverse drug reactions before they occur.
Mechanisms Behind AI Predictive Algorithms
AI predictive algorithms rely on several mechanisms to forecast drug safety issues:
– Data Mining: AI systems mine large datasets to uncover patterns and trends that might indicate potential safety issues. This includes analyzing adverse event databases, patient records, and social media inputs.
– Statistical Modeling: By applying statistical models, AI systems can predict the probability of an adverse drug reaction occurring based on similarity to past events and patient characteristics.
– Risk Assessment Algorithms: AI can evaluate the risk levels of different drug compounds by correlating historical data with new information, helping guide regulatory actions and safety measures.
Improving Accuracy in Predicting Adverse Drug Reactions
AI plays a pivotal role in enhancing the accuracy of predictions related to adverse drug reactions (ADRs):
– Comprehensive Data Integration: By amalgamating disparate data sources, AI offers a holistic view of drug safety, thereby improving predictive accuracy. Sources include clinical data, real-world evidence, and spontaneous reporting systems.
– Continuous Learning and Adaptation: AI models are designed to learn continually from new data, refining their predictions over time. This adaptability means AI tools can increasingly anticipate ADRs with precision.
– Sophisticated Pattern Recognition: Machine learning algorithms excel in identifying complex patterns, uncovering previously unnoticed correlations that contribute to ADR risks.
Real-World Applications of Predictive AI
The application of predictive AI extends into numerous real-world settings, impacting drug safety positively:
– Personalized Medicine: By predicting how individual patients might react to specific drugs, AI fosters the development of personalized treatment plans that minimize risks and enhance efficacy.
– Pre-approval Drug Assessments: During clinical trials, predictive AI helps in assessing the safety profiles of new drugs, improving the likelihood of safe medications reaching the market.
– Post-market Surveillance: In post-marketing, predictive tools continue monitoring drug performance in broader populations, ensuring ongoing safety evaluations and timely action if new risks emerge.
In conclusion, integrating AI in pharmacovigilance is reshaping the landscape of drug safety. With its capabilities in data analysis and predictive modeling, AI not only enhances the detection and prevention of adverse drug reactions but also steers the healthcare sector towards safer and more effective treatment solutions. The future promises even more sophisticated applications, making AI an indispensable ally in advancing global health outcomes.
Future Implications of AI in Pharmacovigilance
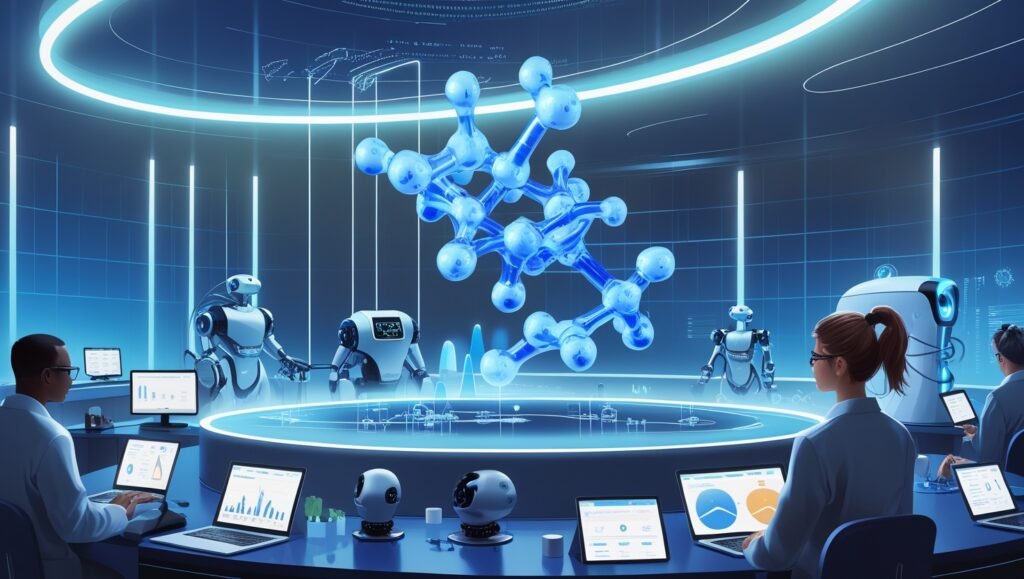
The integration of artificial intelligence into pharmacovigilance isn’t just a trend—it’s a transformative force set to revolutionize how we monitor and ensure drug safety. Imagine a future where potential drug safety issues are flagged long before they pose a threat to patient well-being. This isn’t science fiction; it’s the exciting horizon AI is preparing for us. Let’s explore what this could mean for the future.
Enhanced Predictive Accuracy
One of the most promising aspects of AI in pharmacovigilance is its unparalleled ability to sift through mountains of data and spot patterns that would likely go unnoticed by human analysts. AI algorithms can analyze data points from clinical trials, patient records, and real-world usage, identifying potential safety concerns with pinpoint accuracy. By doing so, AI can improve the early prediction of adverse drug reactions and bolster proactive measures to prevent them.
– Early Detection: Through machine learning, AI systems can identify subtle signals indicative of harmful drug interactions.
– Real-time Analysis: Continuous monitoring ensures that any emerging patterns of concern are caught promptly, reducing the risk of widespread adverse effects.
Streamlined Regulatory Processes
The role of regulatory bodies is crucial in ensuring drug safety, but their processes can often be lengthy and labor-intensive. AI’s efficiency can streamline these processes significantly. By automating routine tasks such as data collection and initial analysis, AI enables human experts to focus on making critical decisions with support from enriched insights.
– Regulatory Assistance: AI tools can prepare robust data analyses for regulatory submissions, expediting approvals without compromising safety.
– Automated Reporting: Integrated AI systems can generate detailed pharmacovigilance reports, ensuring compliance with international safety standards effortlessly.
Global Drug Safety Surveillance
AI’s reach is not limited by geography, making it an invaluable tool for global pharmacovigilance. It can consolidate data from various countries, offering a comprehensive view of a drug’s safety profile worldwide. This global perspective enhances the ability to act swiftly in the face of emerging safety issues that transcend borders.
– Global Data Integration: By pooling data from different regions, AI enhances our understanding of how drugs affect diverse populations.
– Cross-border Collaborations: Facilitating shared insights among international health organizations leads to improved global drug safety standards.
Patient-Centric Care Innovation
AI doesn’t just benefit regulators and healthcare providers; it paves the way for more personalized and safer patient experiences. By leveraging AI, we can inform patients of the specific risks and benefits associated with drugs based on their health profiles.
– Personalized Risk Assessment: AI can deliver targeted safety warnings and guidance to individual patients.
– Improved Healthcare Outcomes: Ultimately, AI leads to better-informed decisions by both clinicians and patients, enhancing overall treatment safety and efficacy.
In conclusion, the future implications of AI in pharmacovigilance promise not only to fortify drug safety but to reimagine it entirely. With advanced technologies paving the way, we stand on the brink of a healthcare revolution, where patient safety is continuously enhanced through smart, data-driven insights. As AI continues to evolve, its integration into pharmacovigilance will empower a healthcare ecosystem that is ever more responsive, effective, and compassionate.
Conclusion
Incorporating artificial intelligence into pharmacovigilance offers exciting possibilities for transforming drug safety. With AI’s ability to rapidly analyze vast datasets and detect patterns, healthcare professionals can predict potential drug safety issues more effectively. As AI tools become more advanced, we can expect enhancements in early warning systems, leading to a safer healthcare environment for everyone. Embracing this technology will not only improve patient outcomes but also streamline the process of drug monitoring and regulation.